´ëÇѾð¾îÇÐȸ ÀüÀÚÀú³Î
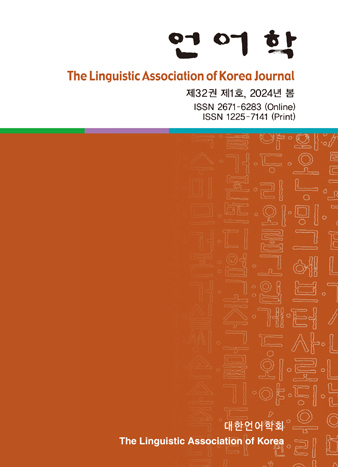
-
ÀΰøÁö´É ÇнÀÀ» À§ÇÑ Áß³ë³âÃþ ¹æ¾ð À½¼º µ¥ÀÌÅÍ ±¸Ãà Àü·« ¹× ºÐ¼®
-
¿¡µàÅ×Å©¸¦ Ȱ¿ëÇÑ ¿µ¾î ¼ö¾÷¿¡ ´ëÇÑ ÃʵîÇлý°ú ÇкθðÀÇ ÀνĿ¡ °üÇÑ ¿¬±¸
-
Çѱ¹¾î¿Í ¿µ¾îÀÇ Â÷ÀÌ¿¡ µû¸¥ ÀÔ·Âó¸® ±³¼ö°¡ Çѱ¹ ÁßÇб³ ÇнÀÀÚµéÀÇ wh-Àǹ®¹® ½Àµæ¿¡ ¹ÌÄ¡´Â ¿µÇâ
-
Adaptation of the English Agentive Suffix in Korean: An Optimality-Theoretic Analysis
-
Assessing Derivational Affix Knowledge among Korean University Learners
32±Ç 1È£ (2024³â 3¿ù)
- ÀΰøÁö´É ÇнÀÀ» À§ÇÑ Áß³ë³âÃþ ¹æ¾ð À½¼º µ¥ÀÌÅÍ ±¸Ãà Àü·« ¹× ºÐ¼®
-
¿Á¼º¼ö ¡¤ ±è¼ö¿¬
Pages : 1-19
Abstract
Keywords
# ÀΰøÁö´É ÇнÀ¿ë µ¥ÀÌÅÍ(AI training data) # ³ëÀÎ À½¼º(elderly speech) # ¹æ¾ð µ¥ÀÌÅÍ(dialect data) # µ¥ÀÌÅÍ ¶óº§¸µ(data labeling) # ¹ßÈ Àǵµ(speech intent) # °¨¼º(emotion)
References
- ·ù¼º±â. (2016). °æ³² Çϵ¿ ¹æ¾ðÀÇ ¹®¹ý ÇüÅÂ¿Í ¼¼´ë ¹× ÀÇ½Ä º¯È¿¡ µû¸¥ ¹®¹ý ÇüÅ »ç¿ë º¯È ¿¬±¸. ±¹Á¦¾ð¾î¹®ÇÐ, 33, 1-37.
- ·ù¼º±â. (2017). ³²¿ø ¹æ¾ð ¹®¹ý ÇüÅÂ¿Í »ç¿ë ¾ç»ó º¯È ¿¬±¸. ±¹Á¦¾ð¾î¹®ÇÐ, 37, 57-94.
- ½É¿ìâ, ÁøÇýºó, ±è¼¼Áø, ±è¼±µ¿. (2023). ARC ¹®Á¦ ÇØ°áÀ» À§ÇÑ ÇÁ·ÒÇÁÆ® ¿£Áö´Ï¾î¸µÀÇ °¡´É¼º. Çѱ¹Á¤º¸°úÇÐȸ Çмú¹ßÇ¥³í¹®Áý, 397-399.
- À̱Ⱙ. (2008). ³óÃÌ Áö¿ªÀÇ ÀÌÁÖ ¿Ü±¹ÀÎ ¿©¼ºµéÀ» À§ÇÑ ¹æ¾ð ±³À°. ÇѱÛ, 280, 165-202.
- À念¿ì, ¼Çϸ°, ¼¿µ±Õ. (2023). ÁÖ¾îÁø ÁÖÁ¦¿¡ ´ëÇÑ ¼Ò¼È¹Ìµð¾î µ¥ÀÌÅͼ »ý¼ºÀ» À§ÇÑ ÆÄÀÌÇÁ¶óÀÎ ¼³°è ¹× ±¸Çö. Çѱ¹Á¤º¸°úÇÐȸ Çмú¹ßÇ¥³í¹®Áý, 438-440.
- Beese, C., Vassileiou, B., Friederici, A. D., & Meyer, L. (2019). Age differences in encoding-related alpha power reflect sentence comprehension difficulties. Frontiers in Aging Neuroscience, 11. https://doi.org/10.3389/fnagi.2019.00183
- Fukuda, M., Nishizaki, H., Iribe, Y., Nishimura, R., & Kitaoka, N. (2020). Improving speech recognition for the elderly: a new corpus of elderly Japanese speech and investigation of acoustic modeling for speech recognition. In Proceedings of the Twelfth Language Resources and Evaluation Conference, 6578–6585, Marseille, France. European Language Resources Association.
- Harnsberger, J. D., Shrivastav, R., Brown, W. S., Rothman, H., & Hollien, H. (2008). Speaking rate and fundamental frequency as speech cues to perceived age. Journal of Voice, 22(1), 58-69.
- Horton, W. S., Spieler, D. H., & Shriberg E. (2010). A corpus analysis of patterns of age-related change in conversational speech. Psychology and Aging, 25(3), 708-713.
- Jakobson, R. (1960). Closing statements: linguistics and poetics. In T. A. Sebeok (Ed.), Style in Language (pp. 350-377). MIT Press.
- Kemper, S., Herman, R., & Lian, C. (2003)., Age differences in sentence production. The Journals of Gerontology: Series B, 58(5), 260–268.
- Khatun, R. & Sarkar, A. (2024). Deep-keyword net: automated English keyword extraction in documents using deep keyword network based ranking. Multimed Tools Applications. https:/doi.org/10.1007/s11042-024- 18110-5
- Linville, S. E. & Rens, J. (2001). Vocal tract resonance analysis of aging voice using long-term average spectra. Journal of Voice, 15(3), 323-330.
- Searle, J. R. (1969). Speech acts: An essay in the philosophy of language. Cambridge University Press.