´ëÇѾð¾îÇÐȸ ÀüÀÚÀú³Î
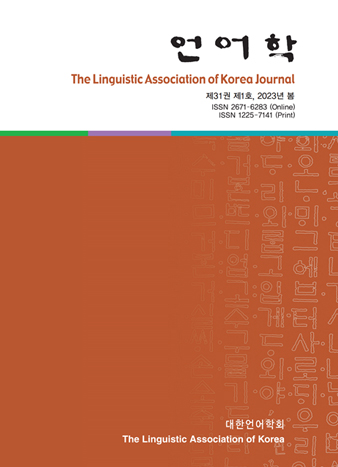
-
21¼¼±â ºñÂ÷º°Àû ¿µ¾î ¾îÈÖÀÇ µîÀå: Bachelorette¿Í IntersexÀ» Áß½ÉÀ¸·Î
-
Àΰ£¹ø¿ª°ú ±â°è¹ø¿ªÀÇ ¿©¼º¾î․³²¼º¾î ºñ±³ ¿¬±¸: ÇÑÀÏ ¹ø¿ªº» 82³â»ý ±èÁö¿µÀÇ ¹®¸»(ÙþØÇ)Ç¥ÇöÀ» Áß½ÉÀ¸·Î
-
Analysis of International Students' Adaptation to Korean Language Environment
-
From Gender-Biased to Gender-Specific and Gender-Inclusive Words: A Corpus-Based Study
31±Ç 1È£ (2023³â 3¿ù)
- Àΰ£¹ø¿ª°ú ±â°è¹ø¿ªÀÇ ¿©¼º¾î․³²¼º¾î ºñ±³ ¿¬±¸: ÇÑÀÏ ¹ø¿ªº» 82³â»ý ±èÁö¿µÀÇ ¹®¸»(ÙþØÇ)Ç¥ÇöÀ» Áß½ÉÀ¸·Î
-
Á¶½Â¿¬
Pages : 45-63
Abstract
Keywords
# ¹®Çбâ°è¹ø¿ª(Literature translation) # ¿©¼º¾î(female language) # ³²¼º¾î(male language) # ÄÚÆÛ½º ºÐ¼®(corpus analysis)
References
- °ûÀº½É. (2014). ùÛ国á³説ªÎìíÜâåÞ翻訳ªË̸ªéªìªëÒ³àõÔôíÞìÑÚªªÎåëç¨Ìºª¤ªË関ª¹ªëìéÍÅóÌ-Ò³ àõ«¸«§«ó«À-øöãÆû¡ãÒªÎÞÅéĪòñéãýªË-. ÀϺ»¹®ÇÐÇк¸, 64, 5-28.
- °ûÀº½É. (2015). ùÛìíÛßæ»â£åöªËªªª±ªëÙþØÇøúúÞªÎò¦ÓôªËμª¹ªëæÚϼ -àõó¬ªòøúª¹ÙþØÇÞòªÎÞÅéÄ ªòñéãýªË-. ÀϺ»¾î±³À°¿¬±¸, 7-28.
- ±è½½±â. (2018). ÀΰøÁö´ÉÀ¸·Î ³¯°³ ´Ü ÀÚµ¿Åë¹ø¿ª ¼ºñ½º. Digieco. KT°æÁ¦°æ¿µ¿¬±¸¼Ò. Retrieved from http://digieco.co.kr/ktfront
- ¸¶½ÂÇý. (2017). ±â°èȵDZ⠾î·Á¿î Àΰ£ ´É·Â°ú ¹®Çйø¿ª ´É·Â ºñ±³ °íÂû ¹× ³íÀÇ. Åë¹ø¿ªÇבּ¸, 21(3), 55-77.
- ¸¶½ÂÇý. (2018). ¹®ÇÐÀÛǰ ±â°è¹ø¿ªÀÇ ÇѰ迡 ´ëÇÑ »ó¼¼ °íÂû. Åë¹ø¿ªÇבּ¸, 22(3), 65-88.
- ¾È¹Ì¿µ. (2020). Çѱ¹¾î¿Í ¿µ¾îÀÇ ¾ð¾îÀû Â÷ÀÌ¿¡ µû¸¥ ±â°è¹ø¿ªÀÇ ¹®Á¦Á¡°ú ±×¿¡ ´ëÇÑ Æ÷½ºÆ® ¿¡µðÆÃ ¹æÇâ Á¦¾È. ¿µ¾î¿µ¹®ÇÐ, 25(1), 103-130.
- ÀÌ»óºó. (2016). Æ®·£½ºÅ©¸®¿¡À̼Ç, ±â°è¹ø¿ª, ¹ø¿ª±³À°ÀÇ ¹Ì·¡. Å뿪°ú ¹ø¿ª, 18(2), 129-152.
- ÀÌ¿ëÈÆ. (2016). RÀ» Ȱ¿ëÇÑ ÄÚÆÛ½º¾ð¾îÇаú Åë°èÇÐ. Çѱ¹¹®È»ç.
- ÀÌÀ¯¾Æ. (2020). ¼Ò¼³ 82³â»ý ±èÁö¿µÀÇ ¹ø¿ª¿¡¼ ³ªÅ¸³ Á¨´õ Ç¥Çö ¾ç»ó¿¡ ´ëÇÑ °íÂû. ÀϺ»¾î¹®ÇÐ, 88(1), 149-178.
- ÀÌÁØÈ£. (2019). ¹®Çйø¿ª Àû¿ëÀ» À§ÇÑ ±â°è¹ø¿ªÀÇ ÇöÁÖ¼Ò. Åë¹ø¿ªÇבּ¸, 23(1): 143-167.
- ÀÌâ¼ö. (2021). ±â°èÇнÀ ¾Ë°í¸®ÁòÀ» Ȱ¿ëÇÑ ¹®Çйø¿ª¿¡¼ÀÇ ±â°è ¹ø¿ª°ú Àΰ£ ¹ø¿ª °á°ú¹° ºÐ·ù ¿¬±¸. ¹ø¿ªÇבּ¸, 22(1), 199-217.
- ÀÌÇØ¹Ì. (2022). ±¹³» ¾ð¾îÇаè¿Í ÀϺ»¾îÇаèÀÇ ±â°è¹ø¿ª °ü·Ã ¿¬±¸ µ¿Ç⠺м®. ÀϺ»¾îÇבּ¸, 71, 125-143.
- ʼº¼÷. (2002). ¹®¸»Ç¥Çö¿¡ °üÇÑ ¿¬±¸. º¸°í»ç, 13-21.
- ÃÖÀ±¿µ. (1996). ±â°è¹ø¿ª°ú ¹®Çйø¿ª. ¹ø¿ª¿¬±¸, 4, 51-61.
- Ȳ¼ö¿µ. (2015). Çѱ¹ÀÎ ÀϺ»¾î ÇнÀÀÚ¿¡ ÀÖ¾î¼ÀÇ ¹®¸»Ç¥Çö¿¡ °üÇÑ ¾ç»ó ¿¬±¸ : Á¾Á¶»ç¿Í
- ÁßµµÁ¾·áÇ¥Çö, Ãß·®Ç¥ÇöÀ» Áß½ÉÀ¸·Î. ÀϺ»¾î±³À°, 72-81
- êóô»éÐ×ì. (2013). 会ü¥ÙþØÇªËªªª±ªë¡¸Ñûª³ªÈªÐ¡¹ªÈ¡¸Ò³ª³ªÈªÐ¡¹ªÎÝÂ×¾-«¸«§«ó«ÀーãÛܬ ÌËú¾ªÈ«¸«§«ó«Àー÷åì¶àõªòò¦øöªÈª·ªÆ-. ìíÜâåުȫ¸«§«ó«Àー, 13, 63-72.
- 奥ï£亜Ú±. (2016). äÆÃ¿¡¼ÀÇ ÀϺ»ÀÎ ¿©¼º Á¨´õÇ¥Çö »ç¿ë°æÇâ¿¡ °üÇÑ ¿¬±¸ –¹®¸»Ç¥ÇöÀ» Áß½ÉÀ¸·Î–. ÀϺ»¾î±³À°¿¬±¸, 139-156.
- á³ô¹ðÄÛÝùê. (2004). ü¥ª·åëç¨ÑûÒ³ó¬-ïÒëù・ëòãÛ・実÷¾£®ìíÜâåުȫ¸«§«ó«Àー, 4, 25-39£®
- ÚÐøýìÎÃ.£¨ 1997).¡¸ Ò³àõ専éÄû¡ãҪΪ¤ªÞ¡¹úÞÓÛìíÜâåÞ研ϼ会øº¡º Ò³àõªÎª³ªÈªÐ£òÅíÞøº£¡»ªÒªÄª¸ßöÛ®.
- ñéÓö悦í (1999). Ò³àõªÎåëç¨・òÅíÞøº¡¡ªÒªÄª¸ßöÛ®.¡¡
- ×ùÙ¥í. (2005). «¸«§«ó«ÀーªËªèªëìíÜâåÞøúúުΫô«¡«ê«¨ー«·«ç«ó£å´Ò´öµªÎéΤªë発ü¥ØÇ ªÎøúúÞû¡ãÒªÈëå声îÜ÷å徴£. EAJS (European Association for Japanese Studies), 43.
- â©ÜâÎÃÚ¸. (2005). «Æ«ì«Ó«É«é«ÞªËªªª±ªëÒ³àõåë稪ȫ¸«§«ó«À-«Õ«£«ë«¿ー. ìíÜâåÞ«¸«§«ó «Àー学会学会ò¼, 5, 23-46.
- â©ÜâÎÃÚ¸. (2007). ñ«íåªÉªÎ強ª¤íÞØüªËªªª±ªëÒ³àõÙþØÇÞòÞÅéÄ-実ð·ªÎ会ü¥ªÈ«É«é«ÞªÈªÎÝïÎò -. 国ð·ÖåÙþð¯çé号, ÝÁÎúñ¶ã¼Ø¡ÓÞ学, 13-22.
- â©ÜâÎÃÚ¸. (2008). «É«é«ÞªËÞŪïªìªëÒ³àõÙþØÇÞò-ÊÅÜâÊ«ªÎëòãÛðàÞÛªèªê. ìíÜâåުȫ¸«§«ó «Àー8, 73-94.
- Hutchins, J. (2005). Current commercial machine translation systems and computer-based translation tools: system types and their uses. International Journal of Translation, 17(1-2), 5-38.
- Toral, A., & Way, A. (2015) ¡®Machine-assisted Translation of Literary Text: A Case Study¡¯, Translation Spaces, 4, 241-268.
- Toral, A., & Way, A. (2018) What level of quality can neural machine translation attain on literary text?. arXiv preprint arXiv, 1801, 04962.
- Voigt, R., & Jurafsky, D. (2012). Towards a literary machine translation: The role of referential cohesion. In Proceedings of the NAACL-HLT 2012 Workshop on Computational Linguistics for Literature, 18-25.