´ëÇѾð¾îÇÐȸ ÀüÀÚÀú³Î
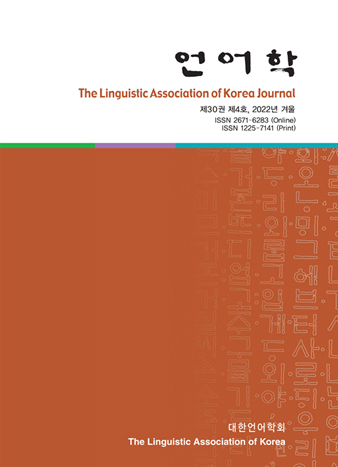
-
Á¶À½°ú ûÃë¿¡ ±Ù°ÅÇÑ ¼ö¾÷À» ÅëÇÑ Çѱ¹ÀÎ ´ëÇлýÀÇ ¿µ¾î¸ðÀ½ ¹ßÀ½ º¯È
-
Çѱ¹¾î ºÎ»ç ºÎÁ¤±Ø¾î ¡®Å롯°ú ¡®¿µ¡¯¿¡ ´ëÇÑ ¿¬±¸: Çѱ¹¾î ±³À°ÀÇ °üÁ¡¿¡¼
-
A Grammar of Contrastive Stripping Construction with Subordinating Conjunctions in English
-
Neural Network Language Models as Psycholinguistic Subjects: Focusing on Reflexive Dependency
-
Authorial Reference and Rhetorical Functions in Research Articles of Mathematics and Linguistics
30±Ç 4È£ (2022³â 12¿ù)
- Neural Network Language Models as Psycholinguistic Subjects: Focusing on Reflexive Dependency
-
Wonil Chung & Myung-Kwan Park
Pages : 169-190
Abstract
Keywords
# reflexive dependency # filler-gap dependency # gender mismatch effect # neural network language model # surprisal
References
- Aurnhammer, C., & Frank, S. (2019). Evaluating information-theoretic measures of word prediction in naturalistic sentence reading. Neuropsychologia, 134, 107-198.
- Bock, K., & Miller, C. A. (1991). Broken agreement. Cognitive Psychology, 23(1), 45-93.
- Chaves, R. P. (2020). What don't RNN language models learn about filler-gap dependencies? Proceedings of the Society for Computation in Linguistics, 3(1), 20-30.
- Chaves, R. P., & Richter, S. N. (2021). Look at that! BERT can be easily distracted from paying attention to morphosyntax. Proceedings of the Society for Computation in Linguistics, 4(1), 28-38.
- Chowdhury, S. A., & Zamparelli, R. 2018. RNN simulations of grammaticality judgments on long-distance dependencies. In Proceedings of the 27th International Conference on Computational Linguistics, 133-144.
- Christiansen, M., & Chater, N. (1999). Connectionist natural language processing: The state of the art. Cognitive Science, 23, 417–37.
- Chung, W., & Park, M.-K. (2018). Are Korean English learners structure-sensitive in reflexive resolution? The Asian International Journal of Life Sciences, 15(4), 2905-2919.
- Da Costa, J., & Chaves, R. (2020). Assessing the ability of Transformer-based Neural Models to represent structurally unbounded dependencies. Proceedings of the Society for Computation in Linguistics, 3(1), 189-198.
- Devlin, J., Chang, M.-W., Lee, K., & Toutanova, K. (2018). Bert: Pre-training of deep bidirectional transformers for language understanding. arXiv preprint arXiv:1810.04805.
- Dillon, B., Mishler, A., Sloggett, S., & Phillips, C. (2013). Contrasting intrusion profiles for agreement and anaphora: Experimental and modeling evidence. Journal of Memory and Language, 69(2), 85-103.
- Elman, J. L. (1990). Finding structure in time. Cognitive Science, 14(2), 179–211.
- Fodor, J., & Pylyshyn, Z. (1988), Connectionism and cognitive architecture: A critical analysis. Cognition, 28, 3-71.
- Frank, M. J., Gagne, C., Nyhus, E., Masters, S., Wiecki, T. V., Cavanagh, J. F., & Badre, D. (2015). fMRI and EEG predictors of dynamic decision parameters during human reinforcement learning. Journal of Neuroscience, 35(2), 485-494.
- Frazier, M., Ackerman, L., Baumann, P., Potter, D., & Yoshida, M. (2015). Wh-filler-gap dependency formation guides reflexive antecedent search. Frontiers in Psychology, 6, 01504.
- Futrell, R., Wilcox, E., Morita, T., & Levy, R. (2018). RNNs as psycholinguistic subjects: Syntactic state and grammatical dependency. arXiv preprint arXiv:1809.01329.
- Goldberg, Y. (2017). Neural network methods for natural language processing. San Francisco, CA: Morgan & Claypool.
- Goodkind, A., & Bicknell, K. (2018). Predictive power of word surprisal for reading times is a linear function of language model quality. In Proceedings of the 8th Workshop on Cognitive Modeling and Computational Linguistics (CMCL 2018), 10-18.
- Gulordava, K., Bojanowski, P., Grave, E., Linzen, T., & Baroni, M. (2018). Colorless green recurrent networks dream hierarchically. arXiv preprint arXiv:1803.11138.
- Hale, J. (2001). A probabilistic Earley parser as a psycholinguistic model. In Second Meeting of the North American Chapter of the Association for Computational Linguistics, 1-8.
- Hao, Y., Mendelsohn, S., Sterneck, R., Martinez, R., & Frank, R. (2020). Probabilistic predictions of people perusing: Evaluating metrics of language model performance for psycholinguistic modeling. arXiv preprint arXiv:2009.03954.
- Hu, J., Gauthier, J., Qian, P., Wilcox, E., & Levy, R. (2020). A systematic assessment of syntactic generalization in neural language models. arXiv preprint arXiv:2005.03692.
- Jäger, L. A., Engelmann, F., & Vasishth, S. (2015). Retrieval interference in reflexive processing: Experimental evidence from Mandarin, and computational modeling. Frontiers in psychology, 6(617).
- Jäger, L. A., Mertzen, D., Van Dyke, J. A., & Vasishth, S. (2020). Interference patterns in subject-verb agreement and reflexives revisited: A large-sample study. Journal of Memory and Language, 111(104063).
- Jozefowicz, R., Vinyals, O., Schuster, M., Shazeer, N., & Wu. Y. (2016). Exploring the limits of language modeling. arXiv 1602.02410.
- Levy, R. (2008). Expectation-based syntactic comprehension. Cognition, 106(3), 1126-1177.
- Lewis, R. L., & Vasishth, S. (2005). An activation‐based model of sentence processing as skilled memory retrieval. Cognitive Science, 29(3), 375-419.
- Linzen, T., & Baroni, M. (2021). Syntactic structure from deep learning. Annual Review of Linguistics, 7, 195-212.
- Linzen, T., Dupoux, E., & Goldberg, Y. (2016). Assessing the ability of LSTMs to learn syntax-sensitive dependencies. Transactions of the Association for Computational Linguistics, 4, 521-535.
- Marvin, R., & Linzen, T. (2018). Targeted syntactic evaluation of language models. arXiv preprint arXiv:1808.09031.
- Mitchell, T. M. (1980). The need for biases in learning generalizations. Proceedings of the 2018 EMNLP Workshop BlackboxNLP: Analyzing and Interpreting Neural Networks for NLP. Rutgers University, New Brunswick, NJ
- Nicol, J., & Swinney, D. (1989). The role of structure in coreference assignment during sentence comprehension. Journal of psycholinguistic research, 18(1), 5-19.
- Peters, M., Neumann, E. M., Iyyer, M., Gardner, M., Clark, C., Lee, K., & Zettlemoyer, L. (2018). Deep contextualized word representations. arXiv:1802.05365.
- Phillips, C. (2006). The real-time status of island phenomena. Language, 82, 795–823.
- Pinker, S., & Prince, A. (1988). On language and connectionism: Analysis of a parallel distributed processing model of language acquisition. Cognition, 28, 73 –193.
- Radford, A., Wu, J., Child, R., Luan, D., Amodei, D., & Sutskever, I. (2019). Language models are unsupervised multitask learners. OpenAI Blog, 1(8), 9.
- Ryu, S. H., & Lewis, R. L. (2021). Accounting for agreement phenomena in sentence comprehension with transformer language models: Effects of similarity-based interference on surprisal and attention. arXiv preprint arXiv:2104.12874.
- Smith, N. J., & Levy, R. (2013). The effect of word predictability on reading time is logarithmic. Cognition, 128(3), 302-319.
- Stowe, L. A. (1986). Parsing WH-constructions: Evidence for on-line gap location. Language and Cognitive Processes, 1(3), 227-245.
- Sturt, P. (2003). A new look at the syntax-discourse interface: The use of binding principles in sentence processing. Journal of Psycholinguistic Research, 32(2), 125-139.
- Sutskever, I., Vinyals, O., & Le, Q. V. (2014). Sequence to sequence learning with neural networks. Advances in Neural Information Processing Systems, 27, 3104– 3112.
- Traxler, M. J., & Pickering, M. J. (1996). Plausibility and the processing of unbounded dependencies: An eye-tracking study. Journal of Memory and Language, 35(3), 454-475.
- Van Schijndel, M., & Linzen, T. (2018). A neural model of adaptation in reading. arXiv preprint arXiv:1808.09930.
- Wilcox, E., Gauthier, J., Hu, J., Qian, P., & Levy, R. (2020). On the predictive power of neural language models for human real-time comprehension behavior. arXiv preprint arXiv:2006.01912.
- Wilcox, E., Futrell, R., & Levy, R. (2021). Using computational models to test syntactic learnability. Proceedings of the Annual Meeting of the Cognitive Science Society, 43, 1-88.
- Wilcox, E., Levy, R., Morita, T., & Futrell, R. (2018). What do RNN language models learn about filler-gap dependencies? arXiv preprint arXiv:1809.00042.
- Xiang, M., Dillon, B., & Phillips, C. (2009). Illusory licensing effects across dependency types: ERP evidence. Brain and Language, 108(1), 40-55.