´ëÇѾð¾îÇÐȸ ÀüÀÚÀú³Î
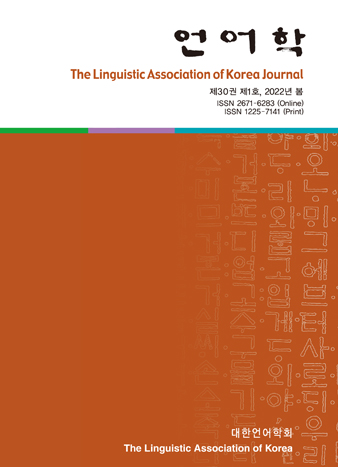
-
°ü°èÀý ºÎÂø ¼±È£¿Í ÁßÀǼº ÀνĿ¡ ³ªÅ¸³ Çѱ¹ÀÎ EFL ÇнÀÀÚµéÀÇ ¿µ¾î ÁßÀÇÀû °ü°èÀý ÇØ¼® ¾ç»ó
-
Àû´ëÀû »ç·Ê¿¡ ±â¹ÝÇÑ ¾ð¾î ¸ðÇüÀÇ Çѱ¹¾î °Ý ±³Ã¼ ÀÌÇØ ´É·Â Æò°¡
-
Çѱ¹¾î ¿¬°á¾î¹Ì ¡°-°í¡±ÀÇ ¹«Ç¥¼º: ¡°¹® ´Ý°í ³ª°¡¡±¸¦ Áß½ÉÀ¸·Î
-
A Case Study of Korean EFL Learners¡¯ Interlanguage in Verb Morphology
-
A Comparative Error Analysis of Neural Machine Translation Output: Based on Film Corpus
-
Lexical Effects in Island Constraints: A Deep Learning Approach
30±Ç 1È£ (2022³â 3¿ù)
- Lexical Effects in Island Constraints: A Deep Learning Approach
-
Yong-hun Lee
Pages : 179-201
Abstract
Keywords
# island constraints # lexical effects # deep learning # BERTLARGE # mixed-effects model
References
- Alexopoulou, T., & Keller, F. (2007). Locality, cyclicity, and resumption: At the interface between the grammar and the human sentence processor. Language, 83(1), 110-160.
- Annamoradnejad, I., & Zoghi, G. (2020). ColBERT: Using BERT sentence embedding for humor detection. arXiv preprint arXiv:2004.12765.
- Baayen, R. (2008). Analyzing linguistic data: A practical introduction to statistics using R. Cambridge: Cambridge University Press.
- Bard, E., Robertson, D., & Sorace, A. (1996). Magnitude estimation of linguistic acceptability. Language, 72, 32-68.
- Barr, D., Levy, R., Scheepers, C., & Tily, H. 2013. Random effects structure for confirmatory hypothesis testing. Journal of Memory and Language, 68, 255-278.
- Chomsky, N. (1973). Conditions on transformations. In A. Stephen & P. Kiparsky (Eds.), A festschrift for Morris Halle (pp. 232-286). New York: Holt, Rinehart and Winston.
- Chomsky, N. (1986). Barriers. Cambridge, MA: MIT Press.
- Chomsky, N. (2000). Minimalist inquiries: The framework. In R. Martin, D. Michaels, & J. Uriagereka (Eds.), Step by step: Essays on minimalist syntax in honor of Howard Lasnik (pp. 89-157.). Cambridge, MA: MIT Press.
- Cowart, W. (1997). Experimental syntax: Applying objective methods to sentence judgments. Thousands Oaks, CA: Sage Publications.
- Devlin, J., Chang, M., Lee, K., & Toutanova, K. (2019). BERT: Pre-training of deep bidirectional transformers for language understanding. arXiv preprint arXiv:1810.04805.
- Goldberg, Y. (2019). Assessing BERT¡¯s syntactic abilities. arXiv preprint arXiv: 1901.05287.
- Goodfellow, I., Bengio, Y., & Courville, A. (2016). Deep learning. Cambridge, MA: MIT Press.
- Gries, S. (2021). Statistics for linguistics with R: A practical introduction (3rd edition). Berlin: Mouton
- Hagstrom, P. (1998). Decomposing questions. Unpublished doctoral dissertation, Massachusetts Institute of Technology.
- Hofmeister, P., & Sag, I. (2010). Cognitive constraints on syntactic islands. Language, 86, 366-415.
- Keller, F. (2000). Gradient in grammar: Experimental and computational aspects of degrees of grammaticality. Unpublished doctoral dissertation, University of Edinburgh.
- Kluender, R. (1998). On the distinction between strong and weak islands: A processing perspective. Syntax and Semantics, 29, 241-279.
- Kluender, R. (2004). Are subject islands subject to a processing account? In V. Chand, A. Kelleher, A. Rodriguez, & B. Schmeiser (Eds.), Proceedings of the west coast conference on formal linguistics 23 (pp. 475-499). Somerville, MA: Cascadilla Press.
- Kluender, R., & Kutas, M. (1993). Subjacency as a processing phenomenon. Language and Cognitive Processes, 8, 573-633.
- Lasnik, H., & Saito, M. (1984). On the nature of proper government. Linguistic Inquiry, 15, 235-289.
- Lee, Y., & Park, Y. (2018). English island constraints by natives and Korean non-natives. The Journal of Studies in Language, 34(3), 439-455.
- Lee, Y. (2016). Corpus linguistics and statistics using R. Seoul: Hankuk Publishing Co.
- Lee, Y. (2021). English island constraints revisited: Experimental vs. deep learning approach. English Language and Linguistics, 27(3), 21-45.
- Levy, R. (2008). Expectation-based syntactic comprehension. Cognition, 106(3), 1126-1177.
- Park, K., Park, M., & Song, S. (2021). Deep learning can contrast the minimal pairs of syntactic data. Linguistic Research, 38(2), 395-424.
- R Core Team. (2022). R: A language and environment for statistical computing. Vienna: R Foundation for Statistical Computing.
- Reinhart, T. (1997). Quantifier scope: How labor is divided between QR and choice functions. Linguistics and Philosophy, 20, 335-397.
- Rizzi, L. (1990). Relativized minimality. Cambridge, MA: MIT Press.
- Ross, J. (1967). Constraints on variables in syntax. Unpublished doctoral dissertation, Massachusetts Institute of Technology.
- Schütze, C. (1996). The empirical base of linguistics: Grammaticality judgments and linguistic methodology. Chicago, IL: University of Chicago Press.
- Sprouse, J, Wagers, M., & Phillips, C. (2012). A test of the relation between working memory capacity and syntactic island effects. Language, 88, 82-123.
- Sprouse, J. 2008. Magnitude estimation and the non-linearity of acceptability judgments. In N. Abner & J. Bishop (Eds.), Proceedings of the 27th west coast conference on formal linguistics (pp. 397-403). Somerville, MA: Cascadilla Proceedings Project
- Sprouse, J., & Hornstein, N. (2013). Experimental syntax and island effects. Cmabridge, MA: Cambridge University Press.
- Szabolcsi, A. 2007. Strong vs. weak islands. In M. Everaert & H. van Riemsdijk (Eds.), The Blackwell companion to syntax (pp. 479-531). Oxford: Blackwell.
- Truswell, R. (2007). Extraction from adjuncts and the structure of events. Lingua, 117, 1355-1377.
- Tsai, D. (1994). On nominal islands and LF extraction in Chinese. Natural Language and Linguistic Theory, 12, 121-175.
- Vaswani, A., Shazeer, N., Parmar, N., Uszkoreit, J., Jones, L., Gomez, A., Kaiser, L., & Polosukhin, I. (2017). Attention is all you need. arXiv preprint arXiv:1706.03762.
- Wang, A., Singh, A., Michael, J., Hill, F., Levy, O., & Bowman, S. 2019. GLUE: A multi-task benchmark and analysis platform for natural language understanding. arXiv preprint arXiv:1804.07461.
- Wang, A., Pruksachatkun, Y., Nangia, N., Singh, A., Michael, J., Hill, F., Levy, O., & Bowman, S. (2020). SuperGLUE: A stickier benchmark for general-purpose language understanding systems. arXiv preprint arXiv:1905.00537.
- Warstadt, A., Singh, A., & Bowman, S. (2019). Neural network acceptability judgments. arXiv preprint arXiv:1805.12471.
- Wilcox, E., Levy, R., & Futrell, R. (2019a). What syntactic structures block dependencies in RNN language models? arXiv preprint arXiv:1905.10431.
- Wilcox, E., Levy, R., & Futrell, R. (2019b). Hierarchical representation in neural language models: Suppression and recovery of expectations. arXiv preprint arXiv:1906.04068.
- Wilcox, E., Levy, R., Morita, T., & Futrell, R. (2018). What do RNN language models learn about filler-gap dependencies? arXiv preprint arXiv:1809.00042.
- Zhu, Y., Kiros, R., Zemel, R., Salakhutdinov, R., Urtasun, R., Torralba, A., & Fidler, S. (2015). Aligning books and movies: Towards story-like visual explanations by watching movies and reading books. arXiv preprint arXiv:1506.06724