´ëÇѾð¾îÇÐȸ ÀüÀÚÀú³Î
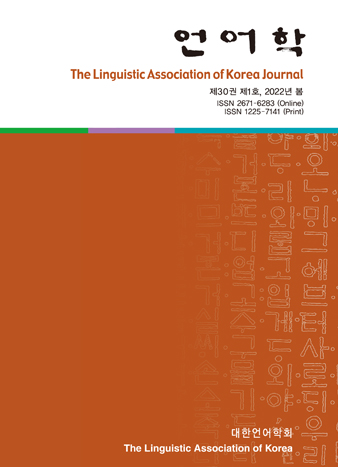
-
°ü°èÀý ºÎÂø ¼±È£¿Í ÁßÀǼº ÀνĿ¡ ³ªÅ¸³ Çѱ¹ÀÎ EFL ÇнÀÀÚµéÀÇ ¿µ¾î ÁßÀÇÀû °ü°èÀý ÇØ¼® ¾ç»ó
-
Àû´ëÀû »ç·Ê¿¡ ±â¹ÝÇÑ ¾ð¾î ¸ðÇüÀÇ Çѱ¹¾î °Ý ±³Ã¼ ÀÌÇØ ´É·Â Æò°¡
-
Çѱ¹¾î ¿¬°á¾î¹Ì ¡°-°í¡±ÀÇ ¹«Ç¥¼º: ¡°¹® ´Ý°í ³ª°¡¡±¸¦ Áß½ÉÀ¸·Î
-
A Case Study of Korean EFL Learners¡¯ Interlanguage in Verb Morphology
-
A Comparative Error Analysis of Neural Machine Translation Output: Based on Film Corpus
-
Lexical Effects in Island Constraints: A Deep Learning Approach
30±Ç 1È£ (2022³â 3¿ù)
- A Comparative Error Analysis of Neural Machine Translation Output: Based on Film Corpus
-
Sungran Koh
Pages : 157-177
Abstract
Keywords
# machine translation # EFL learners # errors # incorrect words # incorrect form
References
- Ali, A. (2016). Exploring the problems of machine translation from Arabic into English language faced by Saudi University student of translation at The Faculty of Arts, Jazan University, Saudi Arabia. IOSR Journal of Humanities and Social Science, 21(4), 55-66. doi: 10.9790/0837-2104025566
- Bojar, O. (2011). Analyzing error types in English-Czech machine translation. The Prague Bulletin of Mathematical Linguistics, 95(1). 63–76. doi: 10.2478/v10108-011-0005-2
- Condon, S., Parvaz, D., Aberdeen, J., Doran, C., Freeman, A., & Awad, M. (2010). Evaluation of machine translation errors in English and IRAQI ARABIC. LREC, European Language Resources Association. doi: 10.21236/ada576234
- Costa, Â., Correia, R., & Coheur, L. (2016). Building a corpus of errors and quality in machine translation: Experiments on error impact. Proceedings of the Tenth International Conference on Language Resources and Evaluation (pp. 288-292). European Language Resources Association (ELRA). http://www.lrec-conf.org/proceedings/lrec2016/pdf/283_Paper.pdf
- Costa, Â., Ling, W., Luís, T., Correia, R., & Coheur, L. (2015). A linguistically motivated taxonomy for machine translation error analysis. Machine Translation, 29(2), 127-161. doi: 10.1007/s10590-015-9169-0
- Dulay, H., Burt, M., & Krashen, S. (1983). Language two. New York, NY: Oxford University Press.
- Emmaanuelle, E.-R., Francic, B.-M., & Eady, S. (2019). SCCOLE: A collaborative platform of error annotation for aligned corpora. 27-35
- Han, C.-H., & Palmer, M. (2005). A morphological tagger for Korean: Statistical tagging combined with corpus-based morphological rule application. Machine Translation, 18(4) 275-297. https://www.jstor.org/stable/20060455
- Lee, I.-C. (Director). (2020). Minari [Movie]. United States: Plan B Entertainment
- Lee, S., & Kim, S. (2018). Pre-editing rules to enhance output quality of machine TRANSLATION: English-Korean and Korean-English. The Journal of Translation Studies, 19(5), 121-154. doi: 10.15749/jts.2018.19.5.005
- Leng, L., & Shan, G. (2019). Analysis and research on lexical errors in machine translation in Chinese and Korean translation. Retrieved from https://francispress.com/index.php/papers/1019
- Llitjós, A., Carbonell, J., & Lavie, A. (2005). A framework for interactive and automatic refinement of transfer-based machine translation. In Proceedings of the 10th Annual Conf. of the European Association for Machine Translation (EAMT), Budapest, Hungary.
- Lyons, S. (2016). Quality of Thai to English machine translation. Retrieved September 16, 2021, from https://link.springer.com/chapter/10.1007/978-3-319-42706-5_20
- Mohamed, A. (2019). Neural and statistical machine translation: A comparative error analysis. 17th International Conference on Translation https://www.researchgate.net/publication/335608441_Neural_and_Statistical_Machine_Translation_A_comparative_error_analysis
- Mohamed, Z. H., & Shafeen N. M. (2017). A brief study of challenges in machine translation. International Journal of Computer Science Issues, 14(2), 54-57. https://doi.org/10.20943/01201702.5457
- Popović, M., & Burchardt, A. (2011). From human to automatic error classification for machine. Retrieved September 20, 2021, from https://www.researchgate.net/publication/270878261_From_human_to_automatic_error_classification_for_machine_translation_output
- Popović, M. & Ney, H. (2007). Word error rates: Decomposition over POS Classes and applications for Error Analysis. In Proceedings of the Second Workshop on Statistical Machine Translation (pp. 48-55). Association for Computational Linguistics.
- Richard, J. (1974). Errors analysis: Perspectives on second language acquisition. London, UK: Longman
- Stankevičiūtė, G., Kasperaviciene, R., Horbacauskiene, J. (2017). Issues in machine translation. International Journal on Language, Literature and Culture in Education, 4(1), 75-88. https://www.semanticscholar.org/paper/Issues-in-Machine-TranslationStankevi%C4%8Di%C5%ABt%C4%97-Kasperaviciene/8c8489bb5e1514931f7284b9b8 9c0236b877a6b7
- Vanjani, M. (2020). A comparison of free online machine language translators. Journal of Management Science and Business Intelligence, 5(1), 26-31.
- Vilar, D., Xu, J., d¡¯Haro, L. F., & Ney, H. (2006). Error analysis of statistical machine translation output. Proceedings of the 5th international Conference on Language Resources and Evaluation (LREC), 697-702.