´ëÇѾð¾îÇÐȸ ÀüÀÚÀú³Î
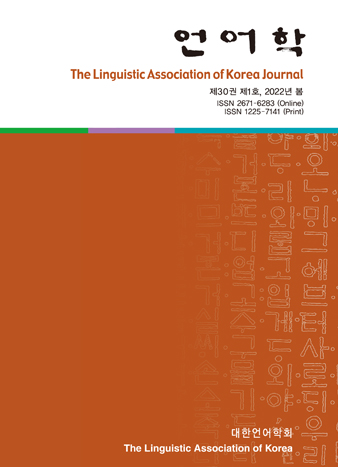
-
°ü°èÀý ºÎÂø ¼±È£¿Í ÁßÀǼº ÀνĿ¡ ³ªÅ¸³ Çѱ¹ÀÎ EFL ÇнÀÀÚµéÀÇ ¿µ¾î ÁßÀÇÀû °ü°èÀý ÇØ¼® ¾ç»ó
-
Àû´ëÀû »ç·Ê¿¡ ±â¹ÝÇÑ ¾ð¾î ¸ðÇüÀÇ Çѱ¹¾î °Ý ±³Ã¼ ÀÌÇØ ´É·Â Æò°¡
-
Çѱ¹¾î ¿¬°á¾î¹Ì ¡°-°í¡±ÀÇ ¹«Ç¥¼º: ¡°¹® ´Ý°í ³ª°¡¡±¸¦ Áß½ÉÀ¸·Î
-
A Case Study of Korean EFL Learners¡¯ Interlanguage in Verb Morphology
-
A Comparative Error Analysis of Neural Machine Translation Output: Based on Film Corpus
-
Lexical Effects in Island Constraints: A Deep Learning Approach
30±Ç 1È£ (2022³â 3¿ù)
- Àû´ëÀû »ç·Ê¿¡ ±â¹ÝÇÑ ¾ð¾î ¸ðÇüÀÇ Çѱ¹¾î °Ý ±³Ã¼ ÀÌÇØ ´É·Â Æò°¡
-
¼Û»óÇå ³ë°»ê ¹Ú±Ç½Ä ½Å¿î¼· Ȳµ¿Áø
Pages : 45-72
Abstract
Keywords
# Àû´ëÀû »ç·Ê(adversarial examples) # °Ý ±³Ã¼(case alternation) # µö·¯´×(deep learning) # °íÀÇÀû ÀâÀ½(intended noise) # °ß°í¼º(robustness) # ¾ð¾î ¸ðÇü(language model) # Æò°¡(evaluation)
References
- ±è¹Ì·É. (2004). °Ý±³Ã¼ ¾ç»ó¿¡ µû¸¥ µ¿»ç ºÐ·ù¿¡ ´ëÇÑ ¿¬±¸. Çѱ¹¾îÇÐ, 25, 161-190. ¹Ú±Ç½Ä, ±è¼ºÅÂ, ¼Û»óÇå. (2021). ÃּҴ븳 ¹®Àå½ÖÀ» Ȱ¿ëÇÑ Çѱ¹¾î »çÀüÇнÀ¸ðµ¨ÀÇ Åë»ç
- ¿¬±¸ Ȱ¿ë °¡´É¼º °ËÁõ. ¾ð¾î¿Í Á¤º¸, 25(3), 1-21.
- ¼Ûâ¼±. (2019). °ÝÁ¶»ç ±³Ã¼ Çö»óÀ» ÅëÇØ º» ±¹¾îÀÇ °Ý ±â´É. ±¹¾î±³À°¿¬±¸, 71, 21-38. ¿ìÇü½Ä. (1996). ±¹¾îÀÇ Å¸µ¿»ç ±¸¹® ¿¬±¸. ¼¿ï: µµ¼ÃâÆÇ ¹ÚÀÌÁ¤.
- À̱ԹÎ, ±è¼ºÅÂ, ±èÇö¼ö, ¹Ú±Ç½Ä, ½Å¿î¼·, ¿Õ±ÔÇö, ¹Ú¸í°ü, ¼Û»óÇå. (2021). DeepKLM - Åë»ç ½ÇÇè À» À§ÇÑ Àü»ê ¾ð¾î¸ðµ¨ ¶óÀ̺귯¸®. ¾ð¾î»ç½Ç°ú °üÁ¡, 52, 265-306.
- ÀÌÁ¾±Ù. (2006). Çѱ¹¾î µ¿»ç¿Í ´ë°Ý¿¡ °üÇÑ ¿¬±¸. ¾ð¾îÇÐ, 14(1), 223-242.
- ÀÌÈ«½Ä. (2004). Á¶»ç ¡®À»¡¯ÀÇ Àǹ̿¡ ´ëÇÏ¿©. Çѱ¹¾î ÀǹÌÇÐ, 15, 303-327.
- Çѱ¹ÀüÀÚÅë½Å¿¬±¸¿ø. (2019). KorBERT(Korean Bidirectional Encoder Representations from Transformers). https://aiopen.etri.re.kr/service_dataset.php.
- È«À缺, À̼ºÇå (2007). ¼¼Á¾ ÀüÀÚ»çÀü : Àü»ê¾îÈֺημÀÇ Æ¯¼º°ú ÀÇÀÇ. Çѱ¹Á¤º¸°úÇÐȸ ¾ð¾î°øÇÐ ¿¬±¸È¸ Çмú¹ßÇ¥ ³í¹®Áý, 323-331.
- Bender, E. M. (2009). Linguistically naïve != language independent: Why NLP needs linguistic typology. Paper presented at the EACL 2009 Workshop on the Interaction between Linguistics and Computational Linguistics: Virtuous, Vicious or Vacuous?, 26-32.
- Da Costa, J. K., & Chaves, R. P. (2020). Assessing the ability of Transformer- based Neural Models to represent structurally unbounded dependencies. Paper presented at the Society for Computation in Linguistics, 3(1), 189-198.
- Devlin, J., Chang, M. W., Lee, K., & Toutanova, K. (2019). BERT: Pre-training of deep bidirectional transformers for language understanding. Paper presented at the 2019 Conference of the North American Chapter of the Association for Computational Linguistics: Human Language Technologies, 4171-4186.
- Ebrahimi, J., Lowd, D., & Dou, D. (2018). On adversarial examples for character- level neural machine translation. Paper presented at the 27th International Conference on Computational Linguistics, 653-663.
- Fukuda, S. (2020). The syntax of variable behavior verbs: Experimental evidence from the accusative–oblique alternations in Japanese. Journal of Linguistics, 56(2), 269-314.
- Garg, S., & Ramakrishnan, G. (2020). BAE: BERT-based adversarial examples for text classification. Paper presented at the 2020 Conference on Empirical Methods in Natural Language Processing, 6174-6181.
- Goldberg, Y. (2019). Assessing BERT's syntactic abilities. arXiv preprint arXiv:1901.05287. Goodfellow, I. J., Shlens, J., & Szegedy, C. (2015). Explaining and harnessing adversarial examples. Paper presented at the ICLR 2015.
- Hale, J. (2001). A probabilistic Earley parser as a psycholinguistic model. Paper presented at the Second meeting of the north American chapter of the association for computational linguistics.
- Hu, J., Gauthier, J., Qian, P., Wilcox, E., & Levy, R. P. (2020). A systematic assessment of syntactic generalization in neural language models. Paper presented at the Association for Computational Linguistics, 1725-1744.
- Jeretic, P., Warstadt, A., Bhooshan, S., & Williams, A. (2020). Are natural language inference models IMPPRESsive? Learning IMPlicature and PRESupposition. Paper presented at the 58th Annual Meeting of the Association for Computational Linguistics, 8690-8705.
- Jiang, N., & de Marneffe, M. C. (2021). He Thinks He Knows Better than the Doctors: BERT for Event Factuality Fails on Pragmatics. Transactions of the Association for Computational Linguistics, 9, 1081-1097.
- Jin, D., Jin, Z., Zhou, J. T., & Szolovits, P. (2020). Is BERT really robust? a strong baseline for natural language attack on text classification and entailment. Paper presented at the AAAI conference on artificial intelligence, 34(5), 8018-8025.
- Lee, S., Jang, H., Baik, Y., Park, S., & Shin, H. (2020). KR-BERT: A small-scale korean-specific language model. arXiv preprint arXiv:2008.03979.
- Levy, R. (2008). Expectation-based syntactic comprehension. Cognition, 106(3), 1126-1177.
- Marvin, R., & Linzen T. (2018). Targeted syntactic evaluation of language models. Paper presented at the 2018 Conference on Empirical Methods in Natural Language Processing, 1192-1202.
- Meister, C., Pimentel, T., Haller, P., Jäger, L., Cotterell, R., & Levy, R. (2021). Revisiting the uniform information density hypothesis. arXiv preprint arXiv:2109.11635.
- Nie, Y., Williams, A., Dinan, E., Bansal, M., Weston, J., & Kiela, D. (2019). Adversarial NLI: A new benchmark for natural language understanding. Paper presented at the 58th Annual Meeting of the Association for Computational Linguistics, 4885-4901.
- Park, K., Park, M.-K., & Song, S. (2021). Deep learning can contrast the minimal pairs of syntactic data. Linguistic Research, 38(2), 395-424.
- Park, S.-H., & Yi, E. (2021). Perception-production asymmetry for Korean double accusative ditransitives. Linguistic Research, 38(1), 27-52.
- Pires, T., Schlinger, E., & Garrette, D. (2019). How multilingual is multilingual BERT?. Paper presented at the 57th Annual Meeting of the Association for Computational Linguistics, 4996-5001.
- Sinha, K., Jia, R., Hupkes, D., Pineau, J., Williams, A., & Kiela, D. (2021). Masked language modeling and the distributional hypothesis: Order word matters pre-training for little. Paper presented at the 2021 Conference on Empirical Methods in Natural Language Processing, 2888-2913.
- Szegedy, C., Zaremba, W., Sutskever, I., Bruna, J., Erhan, D., Goodfellow, I., & Fergus, R. (2014). Intriguing properties of neural networks. Paper presented at International Conference on Learning Representations (ICLR).
- Taylor, W. L. (1953). ¡°Cloze procedure¡±: A new tool for measuring readability. Journalism quarterly, 30(4), 415-433.
- Wei, J., Garrette, D., Linzen, T., & Pavlick, E. (2021). Frequency Effects on Syntactic Rule Learning in Transformers. Paper presented at the 2021 Conference on Empirical Methods in Natural Language Processing, 932-948.
- Wilcox, E., Levy, R., & Futrell, R. (2019). Hierarchical representation in neural language models: Suppression and recovery of expectations. arXiv preprint arXiv:1906.04068.
- Wu, Y., Schuster, M., Chen, Z., Le, Q. V., Norouzi, M., Macherey, W., ... & Dean, J. (2016). Google's neural machine translation system: Bridging the gap between human and machine translation. arXiv preprint arXiv:1609.08144.
- Yanaka, H., & Mineshima, K. (2021). Assessing the Generalization Capacity of Pre-trained Language Models through Japanese Adversarial Natural Language Inference. Paper presented at the Fourth BlackboxNLP Workshop on Analyzing and Interpreting Neural Networks for NLP, 337-349.
- Yu, C., Sie, R., Tedeschi, N., & Bergen, L. (2020). Word frequency does not predict grammatical knowledge in language models. Paper presented at the 2020 Conference on Empirical Methods in Natural Language Processing (EMNLP), 4040-4054.
- Zellers, R., Bisk, Y., Schwartz, R., & Choi, Y. (2018). SWAG: A large-scale adversarial dataset for grounded commonsense inference. Paper presented at the 2018 Conference on Empirical Methods in Natural Language Processing, 93-104.