´ëÇѾð¾îÇÐȸ ÀüÀÚÀú³Î
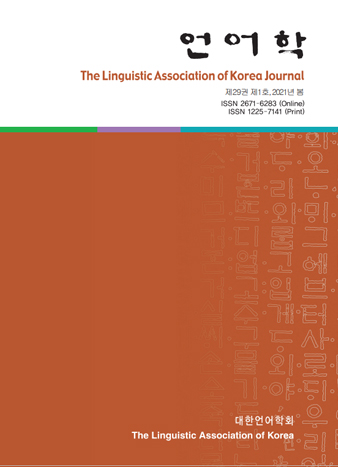
-
COVID-19 ¿µÇâÀ¸·Î ÀÎÇÑ Çѱ¹ À̰ø°è ´ëÇлýÀÇ ¿µ¾î ¿Â¶óÀμö¾÷¿¡ ´ëÇÑ ÀÎ½Ä ¿¬±¸
-
Äڷγª 19·Î ÀÎÇÑ ºñ´ë¸é ¿Â¶óÀÎ °ÀÇ ÀÎ½Ä Á¶»ç: ´ëÇлýÀÇ ¸¸Á·µµ¿Í ºÒ¾Èµµ¸¦ Áß½ÉÀ¸·Î
-
Resolution of Wh/Quantifier Scope Ambiguityin Native and Nonnative Language Processing
-
Current Topics and Controversies in the Analysis of English L2 Learners¡¯ Verb Inflection Errors
29±Ç 1È£ (2021³â 3¿ù)
- Is c-command Machine-learnable?
-
Unsub Shin ¡¤ Myung-Kwan Park ¡¤ Sanghoun Song
Pages : 183-204
Abstract
Keywords
# c-command # deep learning # BERT # surprisal # NPI # reflexive anaphor
References
- Brown, T. B., Mann, B., Ryder, N., Subbiah, M., Kaplan, J., Dhariwal, P., & Amodei, D. (2020). Language models are few-shot learners. Computing Research Repository, arXiv: 2005.14165.
- Chierchia, G. (2013). Logic in grammar: Polarity, free choice, and intervention. Oxford: Oxford University Press.
- Chomsky, N. (1981). Lectures on government and binding. Dordrecht: Foris.
- Clark, K., Khandelwal, U., Levy, O., & Manning, C. D. (2019). What does BERT look at? An analysis of bert's attention. In Proceedings of the 2019 ACL Workshop BlackboxNLP: Analyzing and Interpreting Neural Networks for NLP, 276-286.
- Cunnings, I., Patterson, C., & Felser, C. (2015). Structural constraints on pronoun binding and coreference: Evidence from eye movements during reading. Frontiers in Psychology, 6, 840.
- de Dios-Flores, I., Muller, H., & Phillips, C. (2017). Negative polarity illusions: Licensors that don't cause illusions, and blockers that do. Poster presented at the 30th CUNY conference on human sentence processing, MIT, Cambridge, MA, March 30£April 1.
- Devlin, J., Chang, M. W., Lee, K., & Toutanova, K. (2019). BERT: Pre-training of deep bidirectional transformers for language understanding. In Proceedings of the 2019 Conference of the North American Chapter of the Association for Computational Linguistics: Human Language Technologies, 4171-4186.
- Dillon, B., Mishler, A., Sloggett, S., & Phillips, C. (2013). Contrasting intrusion profiles for agreement and anaphora: Experimental and modeling evidence. Journal of Memory and Language, 69(2), 85-103.
- Ettinger, A. (2020). What BERT is not: Lessons from a new suite of psycholinguistic diagnostics for language models. Transactions of the Association for Computational Linguistics, 8, 34-48.
- Everaert, M. B., Huybregts, M. A., Chomsky, N., Berwick, R. C., & Bolhuis, J. J. (2015). Structures, not strings: Linguistics as part of the cognitive sciences. Trends in Cognitive Sciences, 19(12), 729-743.
- Futrell, R., Wilcox, E., Morita, T., Qian, P., Ballesteros, M., & Levy, R. (2019). Neural language models as psycholinguistic subjects: Representations of syntactic state. In Proceedings of the 18th Annual Conference of the North American Chapter of the Association for Computational Linguistics: Human Language Technologies, 32-42.
- Hewitt, J., & Manning, C. D. (2019). A structural probe for finding syntax in word representations. In Proceedings of the 2019 Conference of the North American Chapter of the Association for Computational Linguistics: Human Language Technologies, 1, 4129-4138.
- Hu, J., Gauthier, J., Qian, P., Wilcox, E., & Levy, R. P. (2020). A systematic assessment of syntactic generalization in neural language models. In Proceedings of the Association for Computational Linguistics, 1725–1744.
- Jumelet, J., & Hupkes, D. (2018). Do language models understand anything? on the ability of LSTMs to understand negative polarity items. In Proceedings of the 2018 EMNLP Workshop BlackboxNLP: Analyzing and Interpreting Neural Networks for NLP, 222–231
- Kim, K.-S. (2010). Is binding possible without c-commanding? The Journal of Studies in Language, 25(4), 675-696.
- Klima, E. S. (1964). Negation in English. In J. A. Fodor & J. J. Katz (Eds.), The structure of language (pp. 246-323). New Jersey: Prentice-Hall.
- Kush, D., Lidz, J., & Phillips, C. (2015). Relation-sensitive retrieval: Evidence from bound variable pronouns. Journal of Memory and Language, 82, 18-40.
- Ladusaw, W. A. (1979). Negative polarity items as inherent scope relations. Unpublished doctoral dissertation, University of Texas at Austin.
- Levy, R. (2008). Expectation-based syntactic comprehension. Cognition, 106(3), 1126-1177.
- Lin, Y., Tan, Y. C., & Frank, R. (2019). Open sesame: Getting inside BERT¡¯s linguistic knowledge. In Proceedings of the 2019 ACL Workshop BlackboxNLP: Analyzing and Interpreting Neural Networks for NLP, 241-253
- Linzen, T. (2019). What can linguistics and deep learning contribute to each other? Response to Pater. Language, 95(1), 99-108.
- Liu, N. F., Gardner, M., Belinkov, Y., Peters, M. E., & Smith, N. A. (2019). Linguistic knowledge and transferability of contextual representations. In Proceedings of the Conference of the North American Chapter of the Association for Computational Linguistics: Human Language Technologies, 1073-1094.
- Liu, Y., Ott, M., Goyal, N., Du, J., Joshi, M., Chen, D., Levy, O., Lewis, M., Zettlemoyer, L., & Stoyanov, V. (2019). RoBERTa: A robustly optimized BERT pretraining approach. Computing Research Repository, arXiv: 1907.11692.
- Marvin, R., & Linzen, T. (2018). Targeted syntactic evaluation of language models. In Proceedings of the 2018 Conference on Empirical Methods in Natural Language Processing, 1192-1202.
- Parker, D., & Phillips, C. (2016). Negative polarity illusions and the format of hierarchical encodings in memory. Cognition, 157, 321-339.
- Reinhart, T., & Reuland, E. (1993). Reflexivity. Linguistic Inquiry, 24(4), 657-720.
- Robinson, D., Gomez, M., Demeshev, B., Menne, D., Nutter, B., & Luke, J. (2017). Broom: Convert statistical analysis objects into tidy data frames. R package version 0.4(2).
- Smith, N. J., & Levy, R. (2013). The effect of word predictability on reading time is logarithmic. Cognition, 128(3), 302-319.
- Taylor, W. L. (1953). ¡°Cloze procedure¡±: A new tool for measuring readability. Journalism Quarterly, 30(4), 415-433.
- Vasishth, S., Brüssow, S., Lewis, R. L., & Drenhaus, H. (2008). Processing polarity: How the ungrammatical intrudes on the grammatical. Cognitive Science, 32(4), 685-712.
- Vaswani, A., Shazeer, N., Parmar, N., Uszkoreit, J., Jones, L., Gomez, A. N., & Polosukhin, I. (2017). Attention is all you need. In Advances in neural information processing systems, 5998-6008.
- Wickham, H. (2017). Tidyverse: Easily install and load the ¡®tidyverse¡¯. R package version 1(1).
- Wilcox, E., Levy, R., Morita, T., & Futrell, R. (2018). What do RNN language models learn about filler-gap dependencies? In Proceedings of the 2018 EMNLP Workshop BlackboxNLP: Analyzing and Interpreting Neural Networks for NLP, 211-221.
- Winter, B. (2019). Statistics for linguists: An introduction using R. London: Routledge.
- Xiang, M., Dillon, B., & Phillips, C. (2009). Illusory licensing effects across dependency types: ERP evidence. Brain and Language, 108(1), 40-55.